The impact of AI copilot on developers productivity
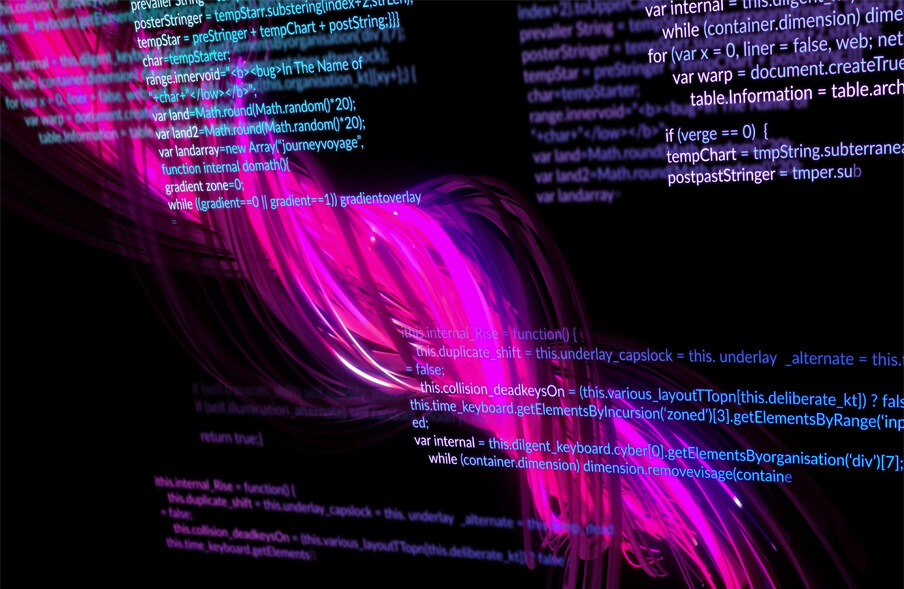
The impact of AI copilot on developers productivity Follow on: In software development, developers often get lost in a whirlwind of repetitive tasks, significantly impacting the time they actually spend writing code. Project teams need advanced tools with machine learning (ML), predictive analytics, and natural language processing (NLP) capabilities that can augment human skills, automate […]
The future of human-AI Interaction: The role of virtual avatars and VR

The future of human-AI Interaction: The role of virtual avatars and VR Follow on: Artificial Intelligence (AI) is redefining human-machine interactions — evolving from rule-based chatbots to context-aware virtual assistants. More recently, generative AI (GenAI) solutions have enabled businesses to hyper-personalize products and boost real-time virtual assistance. McKinsey has found that over 80% of customer […]
Unleashing the potential of XAI: Maximizing GenAI benefits and reducing risks

Unleashing the potential of XAI: Maximizing GenAI benefits and reducing risks Follow on: AI has permeated every part of our lives, evolving from recognizing patterns to achieving human efficiency. Treading deeper into the AI landscape, generative AI (GenAI) has become the new normal, reshaping every industry. Research estimates that GenAI could contribute between $2.6 trillion […]
Transforming Customer Support with AI in Contact Centers

Transforming Customer Support with AI in Contact Centers Follow on: The Road to AI Can AI do to contact centers what it did to Industry 4.0? All industry use cases and market predictions point in the direction of AI-driven contact centers — as the next strategic step for boosting agent productivity, supercharging customer experience, and […]
The Generative AI Evolution: Emerging Trends and Applications Across Industries

The Generative AI Evolution: Emerging Trends and Applications Across Industries Follow on: “It’ll be unthinkable not to have intelligence integrated into every product and service. It’ll just be an expected, obvious thing.” – Sam Altman, co-founder and CEO of OpenAI. Generative AI (GenAI) has expanded the horizons of innovation and challenged us to rethink the […]
Navigating beyond Generative AI: The dawn of hyper-intelligent systems

Navigating beyond Generative AI: The dawn of hyper-intelligent systems Follow on: In the past year, Generative AI (GenAI) has emerged as one of the most remarkable breakthroughs, triggering a transformative wave across the global economic and IT landscape. From redefining customer engagement and reshaping product development to inspiring innovative shifts in business models, GenAI has […]
Top Emerging Trends for AI Platforms in 2024 and Beyond

Top Emerging Trends for AI Platforms in 2024 and Beyond Follow on: “It’s hard to overstate how big of an impact AI and machine learning will have on society over the next 20 years” – Jeff Bezos. In this age and probably in the next century, artificial intelligence (AI) will be the cornerstone for futuristic […]
Unraveling the tapestry: The imperative of human valuation in guiding LLM’s decision-making

Unraveling the tapestry: The imperative of human valuation in guiding LLM’s decision-making Follow on: Introduction In the dynamic landscape of artificial intelligence, Large Language Models (LLMs) stand as formidable entities, capable of processing vast amounts of information and making decisions that impact users. However, the allure of these models also brings forth ethical considerations, especially […]
Decoding the future of Retail Media Networks

Decoding the future of Retail Media Networks Follow on: The ever-evolving retail landscape amid the upsurge of transformative technologies such as cloud computing, Generative AI, Big Data, and the Internet of Things (IoT) has spurred enterprises to reimagine their marketing and advertising tactics. Moreover, shifting channel preferences as consumers move online has led to an […]
Combining low code with emerging AI technologies: Can users truly create compelling apps?

Combining low code with emerging AI technologies: Can users truly create compelling apps? Follow on: Enterprises are no strangers to disruptions, with uncertainty lurking around every corner. In this dynamic environment, adaptability and resilience aren’t just admirable qualities but essential for business survival. The recent geopolitical and economic unpredictability combined with the need to do […]